10 Crucial Considerations on AI Ethics Bias and Transparency You Need to Know
- Paolo Casula
- 5 days ago
- 4 min read
As artificial intelligence (AI) takes a larger role in our everyday lives, the conversation about its ethical implications is becoming more urgent. The presence of AI in decision-making processes raises important questions about bias, transparency, and accountability. This post highlights ten essential considerations related to AI ethics, focusing especially on bias and transparency.
Understanding AI Bias
AI bias occurs when an AI system produces results that are unfairly skewed due to incorrect assumptions in the machine learning process. This can stem from flawed data, biased algorithms, or improper training protocols.
One striking example is hiring algorithms trained on historical data that favor male candidates. In 2018, it was reported that a recruiting tool by a leading tech company had a clear bias against women, resulting in reduced hiring rates for female applicants by nearly 20%. Such systemic biases can have severe consequences, affecting hiring practices and even law enforcement decisions.
Sources of Bias in AI
Identifying sources of bias is crucial for resolving these issues. The origins of bias often stem from:
Historical Disparities: Data reflecting past injustices can lead to discriminatory outcomes in AI systems.
Human Biases: Developers' preconceived notions may inadvertently influence algorithm design.
For instance, research shows that AI trained on public datasets can reflect societal inequalities. A study found that AI trained on datasets of facial images misidentified Black individuals 34% more often than white individuals. Addressing these origins is vital for developing fair AI systems.
Impact of Bias on Society
The effects of AI bias can have significant consequences for social equity. In critical areas like healthcare, finance, and the judicial system, biased outputs can lead to negative outcomes that disproportionately affect marginalized communities.
For example, a 2019 study indicated that an AI system used in healthcare misdiagnosed conditions for Black patients 30% more often than for white patients, leading to harmful health disparities. Such examples highlight the urgent need to address AI bias in order to promote fairness and equity across various sectors.
The Importance of Transparency
While managing bias is vital, promoting transparency in AI systems is equally essential. Transparency means providing clear insights into the algorithms and data used in AI models.
When these systems operate as "black boxes," it becomes difficult for users to trust their functionalities. A report from the Algorithmic Justice League found that 70% of users expressed distrust in AI systems that lack explanation regarding their decision-making processes. Without transparency, the risks of bias are magnified.
Enhancing AI Transparency
Improving transparency requires actionable steps to make AI systems easier to understand. Consider these approaches:
Documenting Data Sources: Clearly outline data used in training models to identify possible biases.
Simplifying Explanations: Offer straightforward, user-friendly explanations of how AI models operate.
By adopting these practices, developers can create a more transparent AI landscape that fosters trust and confidence among users.
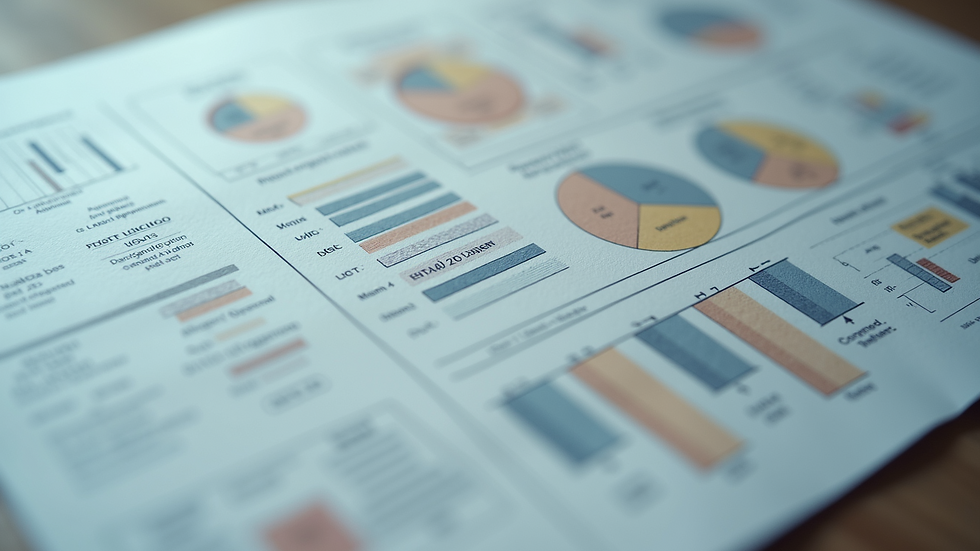
Stakeholder Accountability
Accountability in AI is complex. Developers, organizations, and policymakers must accept responsibility for the systems they create and implement.
Establishing mechanisms for holding stakeholders accountable is essential. This may involve creating regulatory frameworks or third-party audits to assess AI systems for both bias and transparency. According to a 2022 survey, over 60% of organizations that implemented audits noticed a significant decrease in biased outputs.
Promoting Fairness and Equity
Achieving fairness in AI demands a commitment to unbiased data and just outcomes. Equitable AI systems should not only aim to correct past injustices but also work proactively toward a fairer future.
Involving diverse teams in the AI development process is beneficial. Companies that prioritize inclusivity report a marked decrease in biases, as they incorporate varied perspectives that reflect the needs of a broad audience.
The Role of Regulation
Regulatory frameworks are vital for ensuring ethical AI practices. Policymakers must develop guidelines that enforce transparency and address bias within AI systems.
Given that regulations can differ vastly across jurisdictions, global cooperation is necessary to establish standardized ethical practices. This collaboration can lead to fair competition and a unified approach to AI ethics worldwide.
The Future of AI Ethics
As technology evolves, so must our approach to AI ethics. Continuous research and robust dialogue are crucial to understanding the implications of AI in society.
Emerging trends, such as Explainable AI (XAI), focus on making AI more interpretable. In the coming years, we are likely to see the development of new methods for actively combating biases and enhancing accountability in AI.
Moving Forward in AI Ethics
AI ethics is a complex issue, intricately linked to both bias and transparency. As AI technologies increasingly influence our lives, it is essential for stakeholders to address ethical concerns in order to prevent the perpetuation of societal inequalities.
By understanding the sources of bias, supporting transparency, promoting accountability, and pushing for fairness, we can shape an AI landscape that serves everyone equitably.
In a world growing ever more dependent on AI, fostering discussions around ethics, bias, and transparency is critical for building a fairer and more just society.
Comentários